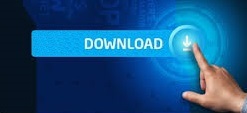
The project will take a few minutes to import. Verify the project name and location, and then select Finish.
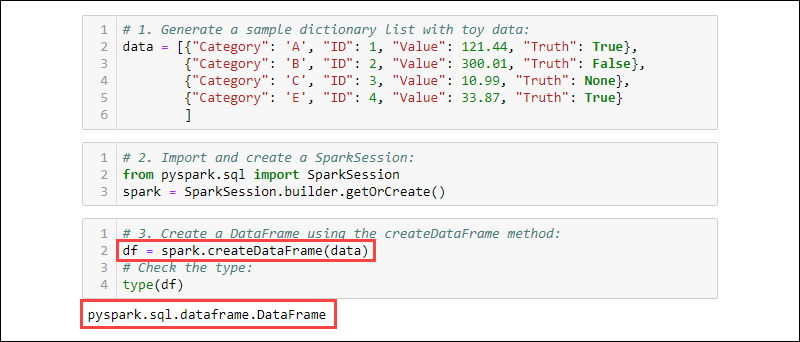
Verify the settings and then select Next. The following values are used in this tutorial: Provide relevant values for GroupId, and ArtifactId. This archetype creates the right directory structure and downloads the required default dependencies to write Scala program.Įxpand Artifact Coordinates. Select the Create from archetype checkbox.įrom the list of archetypes, select :scala-archetype-simple. and navigate to the Java installation directory. This example uses Spark 2.3.0 (Scala 2.11.8). If the Spark cluster version is earlier than 2.0, select Spark 1.x. The creation wizard integrates the proper version for Spark SDK and Scala SDK. This field will be blank on your first use of IDEA. In the New Project window, provide the following information: Property
#Download spark csv jar install#
Select Install for the Scala plugin that is featured in the new window.Īfter the plugin installs successfully, you must restart the IDE. On the welcome screen, navigate to Configure > Plugins to open the Plugins window. See Installing the Azure Toolkit for IntelliJ.ĭo the following steps to install the Scala plugin: This article uses IntelliJ IDEA Community 2018.3.4.Īzure Toolkit for IntelliJ. This tutorial uses Java version 8.0.202.Ī Java IDE. For instructions, see Create Apache Spark clusters in Azure HDInsight. Use IntelliJ to develop a Scala Maven applicationĪn Apache Spark cluster on HDInsight.Maximum number of retries while waiting for the spark-submit OS process to complete.
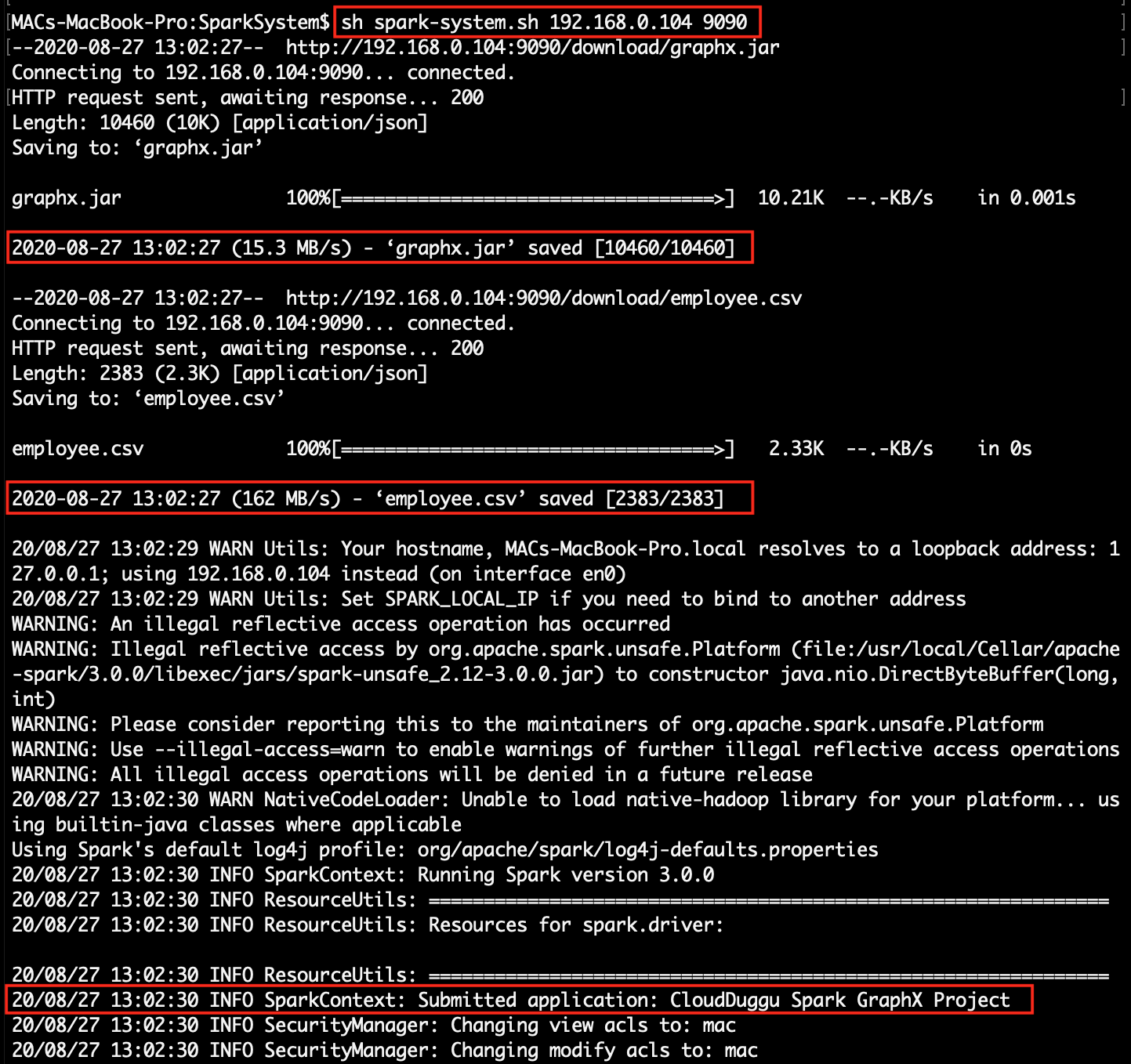
Spark-history-server-polling-persist-after-retries Maximum number of retries while waiting for the Spark History Server to make the Spark Event Logs available. Timeout in seconds used for REST calls on Spark History Server. Spark-webui-polling-persist-after-retries Time in seconds between two polls on the Spark WebUI. Timeout in second used for REST calls on Spark WebUI. Spark-webui-startup-polling-persist-after-retries Maximum number of retries while waiting for the Spark WebUI to come-up.ĭisplays the time in seconds between retries. Table 7-3 Extra Spark Streaming Properties Key SYNCHRONOUS: Spark application is submitted and monitored through OdiOSCommand.ĪSYNCHRONOUS: Spark application is submitted asynchronously through OdiOSCommand and then monitored through Spark REST APIs. If there is no checkpoint, it will start normally.ĭisplays the duration in seconds of a streaming interval.ĭisplays the time in seconds and sets the Spark Streaming context to remember RDDs for this duration.ĭisplays the timeout in seconds before stopping a Streaming application. If set to false, the Spark Streaming application will ignore any existing checkpoints. If set to true, the Spark Streaming application will restart from an existing checkpoint. Every mapping under this base directory will create a sub-directory.Įxample: hdfs://cluster-ns1/user/oracle/spark/checkpoints This property defines the base directory for checkpointing. Table 7-2 Spark Streaming DataServer Properties Key Default value is a pyspark defined hash function portable_hash, which simply computes a hash base on the entire RDD row. Partition Function: User defined partition Lambda function. Partition Keys: User defined partition keys represented as a comma separated column list. Partitions Sort Order: Ascending or descending. Sort Partitions: If this option is set to true, partitions are sorted by key and the key is defined by a Lambda function.

When the default value is set, will be used to invoke the repartition() function. Level of Parallelism: Number of partitions and the default is 0.

Repartition: If this option is set to true, repartition is applied after the transformation of component. ODI has Spark base KM options which let you decide whether and where to do repartitioning. Repartitioning can be done in any step of the whole process, even immediately after data is loaded from source or after processing the filter component. In such cases, the RDD.repartition() api can be used to change the number of partitions. The platform resource is not fully used if the platform that runs the Spark application has more available slots for running tasks than the number of partitions loaded. If the source is a HDFS file, the number of partitions is initially determined by the data block of the source HDFS system.
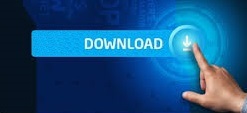